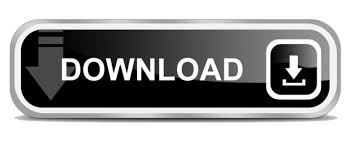
The method of weighted least squares can be used when the ordinary least squares assumption of constant variance in the errors is violated (which is called heteroscedasticity). The method of ordinary least squares assumes that there is constant variance in the errors (which is called homoscedasticity).

This approach uses the framework of generalized linear models, which we discuss in Lesson 12.
:max_bytes(150000):strip_icc()/dotdash_Final_Exploring_the_Exponentially_Weighted_Moving_Average_Nov_2020-04-b65a26eed33f451ba51fabe7298561a7.jpg)
A generalization of weighted least squares is to allow the regression errors to be correlated with one another in addition to having different variances.This leads to weighted least squares, in which the data observations are given different weights when estimating the model – see below. Weight the variances so that they can be different for each set of predictor values.We explored this in more detail in Lesson 7. Apply a variance-stabilizing transformation to the response variable, for example a logarithmic transformation (or a square root transformation if a logarithmic transformation is "too strong" or a reciprocal transformation if a logarithmic transformation is "too weak").Some remedies for refining a model exhibiting excessive nonconstant variance includes the following: For example, if the residual variance increases with the fitted values, then prediction intervals will tend to be wider than they should be at low fitted values and narrower than they should be at high fitted values. MAD - Mean Absolute Deviation, the average of the absolute distances from the average.Excessive nonconstant variance can create technical difficulties with a multiple linear regression model. In the following example, 'high' and 'low' are only relative. This may be good when the outlier is correct, a higher weight emphasizes that the data may spread more, but it may be worse when the outlier is incorrect, See example, and the large weight of the incorrect observation influences more the result.Įxample - Low and High Standard deviation Absolute differences grant equal weight to any difference while square differences grant larger weight to a big difference. (squares is continuously differentiable, easy to derive for a minimum or maximum, while absolute is not).Ģ. In Mathematics it is more elegant to use second power and square root functions than to use the absolute function, hence easier calculation. In other words, why do we use the standard deviation instead of the MAD?ġ. Why does the standard deviation formula use squares differences instead of absolute differences? MAD (Mean Absolute Deviation): The average of the absolute differences.
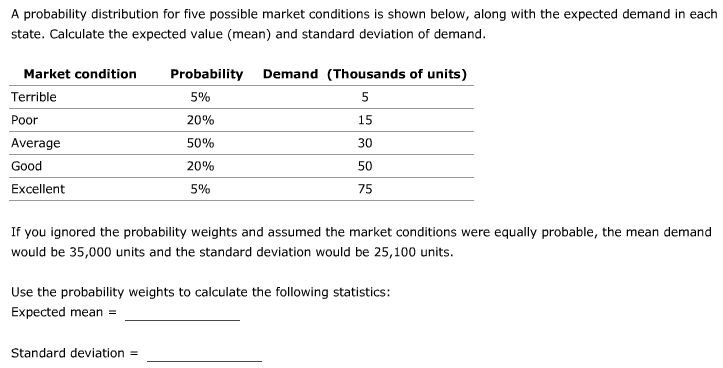
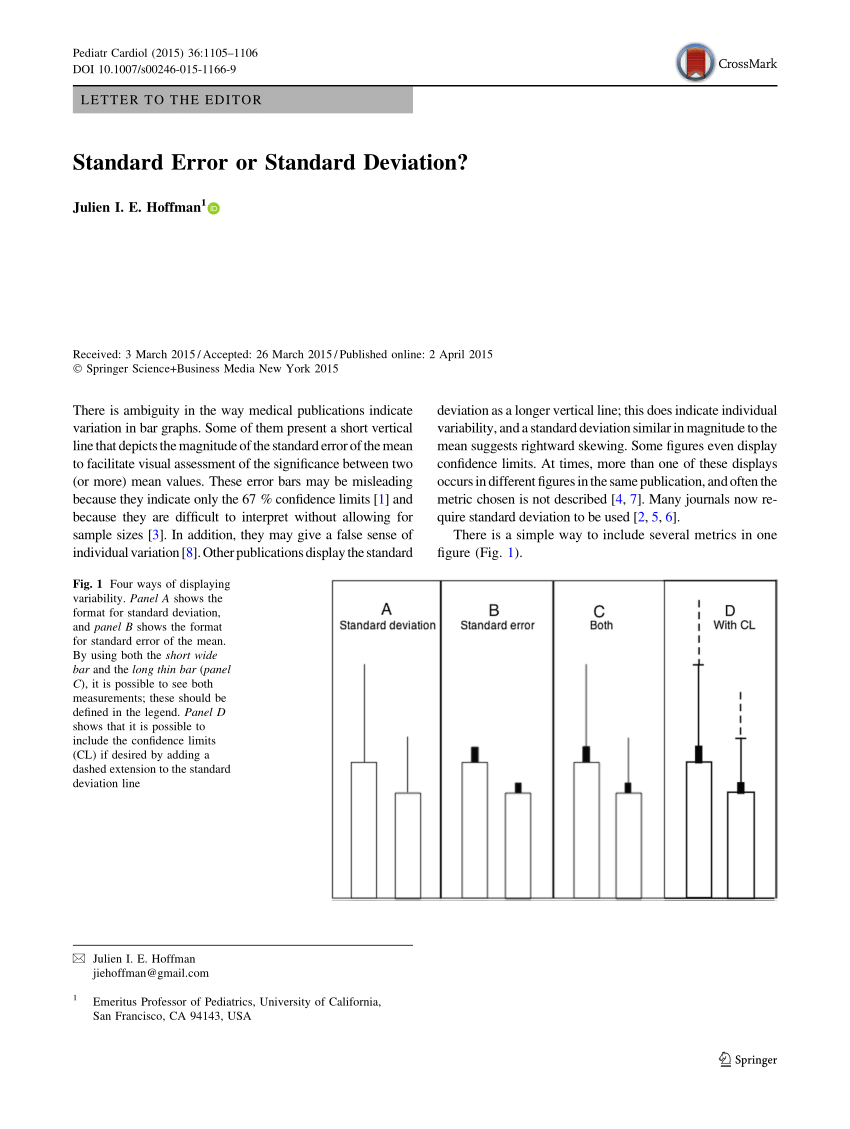
Range: Maximum minus minimum: Max(𝑥 i)-Min(𝑥 i).Ģ.
WEIGHTED STANDARD DEVIATION STATS HOW TO
If you ask a school kid how to measure the variability, he will probably suggest one of the following:ġ. We use n-1 instead of n, to correct the biased estimation of the variance (partially correct the estimation of the standard deviation) ( Bessel's correction). Sample variance formula Sample variance = S 2 = Σ(𝑥i-x̄) 2 n-1 Variance formula Variance = σ 2 = Σ(𝑥i-x̄) 2 nWhen you use a sample to estimate the population's standard deviation you should use the following formula. It is derived from the square root of the distances between each value in the population and the population's mean squared. The standard deviation is a statistic that measures the data variability. Video guide Standard deviation calculator Average calculator Mean Median Mode Q1/Q3/IQR calculator
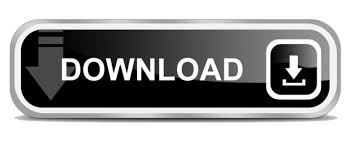